Server-side experiments
Server-side experiments let you use Intempt’s A/B testing while fully controlling how the experiments are rendered on your site. Variations of your custom Server-side experiments are JSON files, so each call results in a JSON that you can use to render an element on your site.
After providing a descriptive name for the experiment, you will be prompted to select the Experiment type: either Client-side or Server-side. Select the Server-side option.
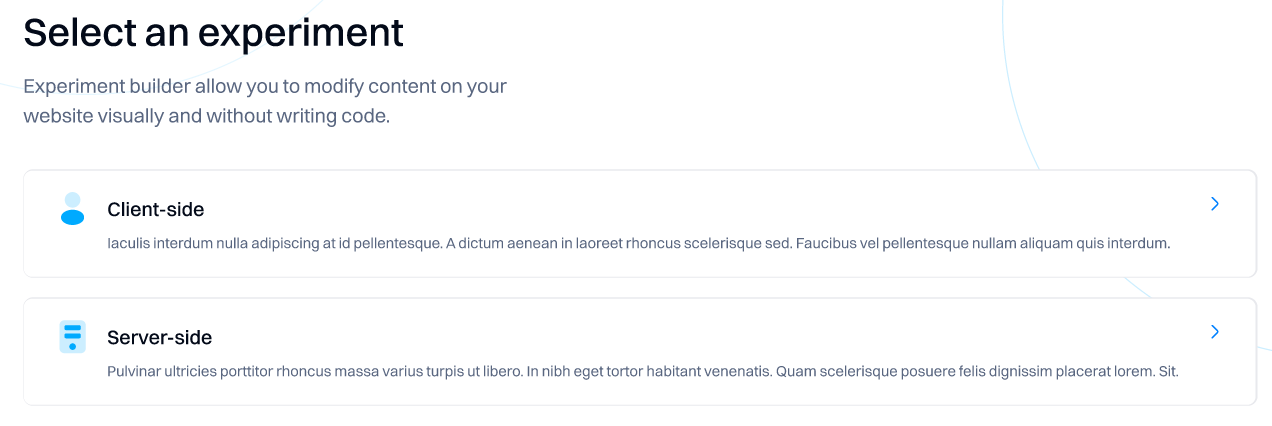
Once the experiment has been named, you will be directed to the Experiment itself where you need to add and configure the experiment variants. By clicking on the Add new variant you will be presented with the variants templates. You can either choose one of the suggested templates or create one by yourself.
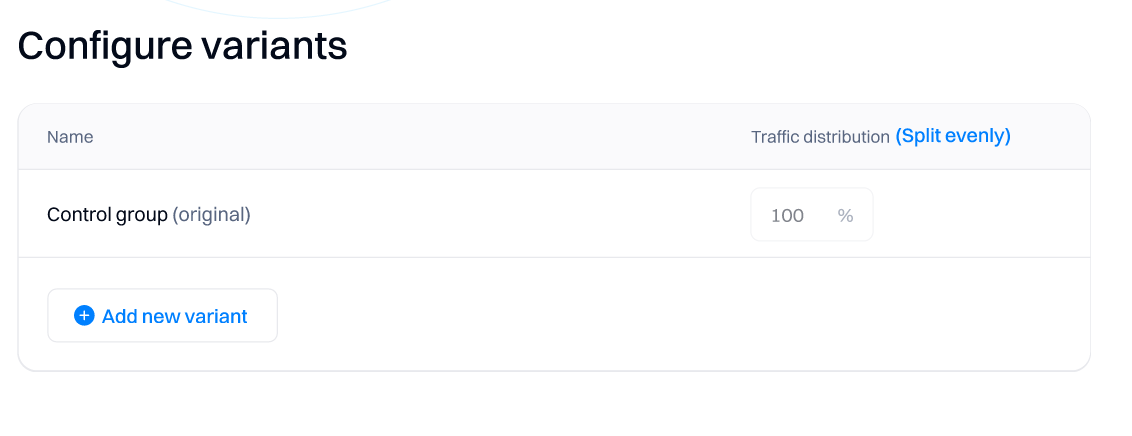
Enter a JSON payload variation for a selected section in your site.
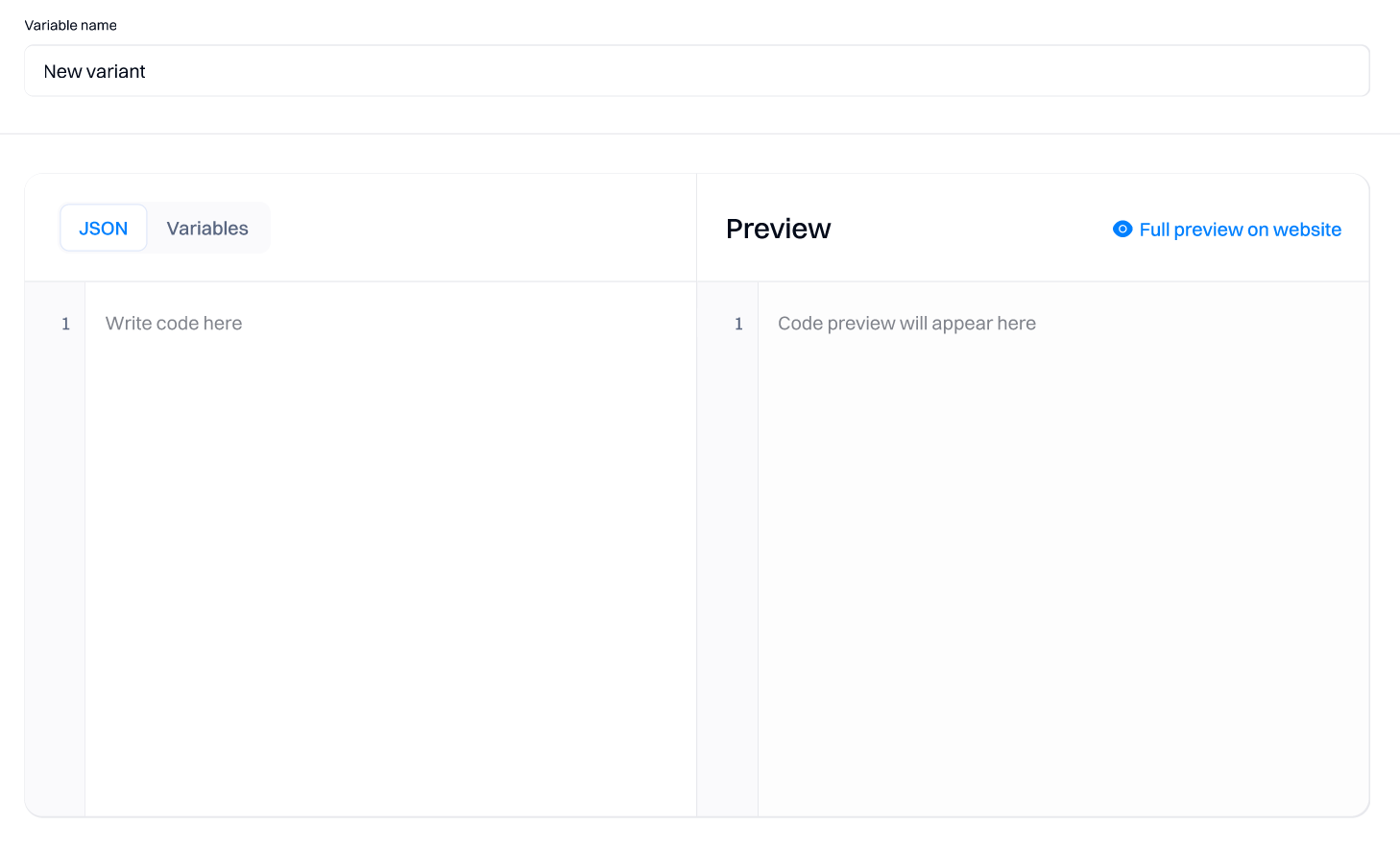
If you click on the Variables option you can enter the values for the added payload.
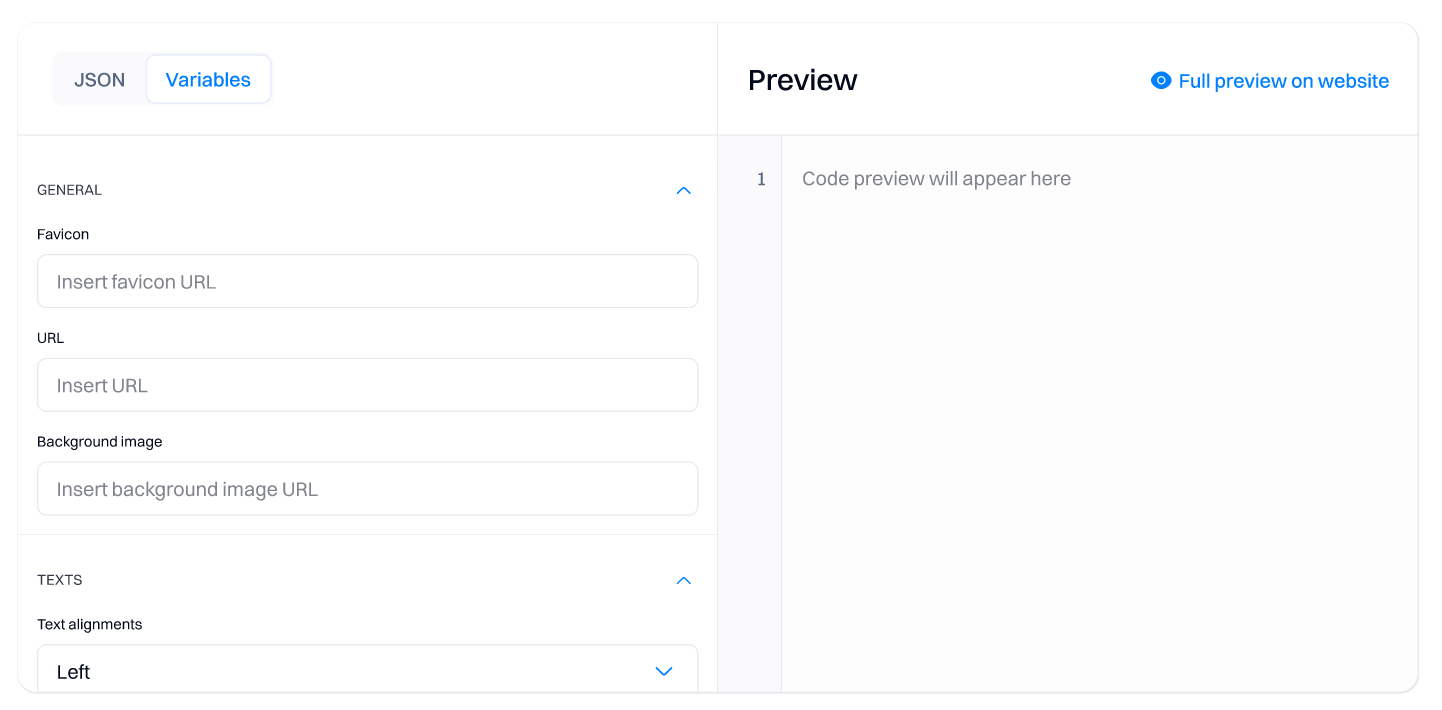
Updated 3 months ago